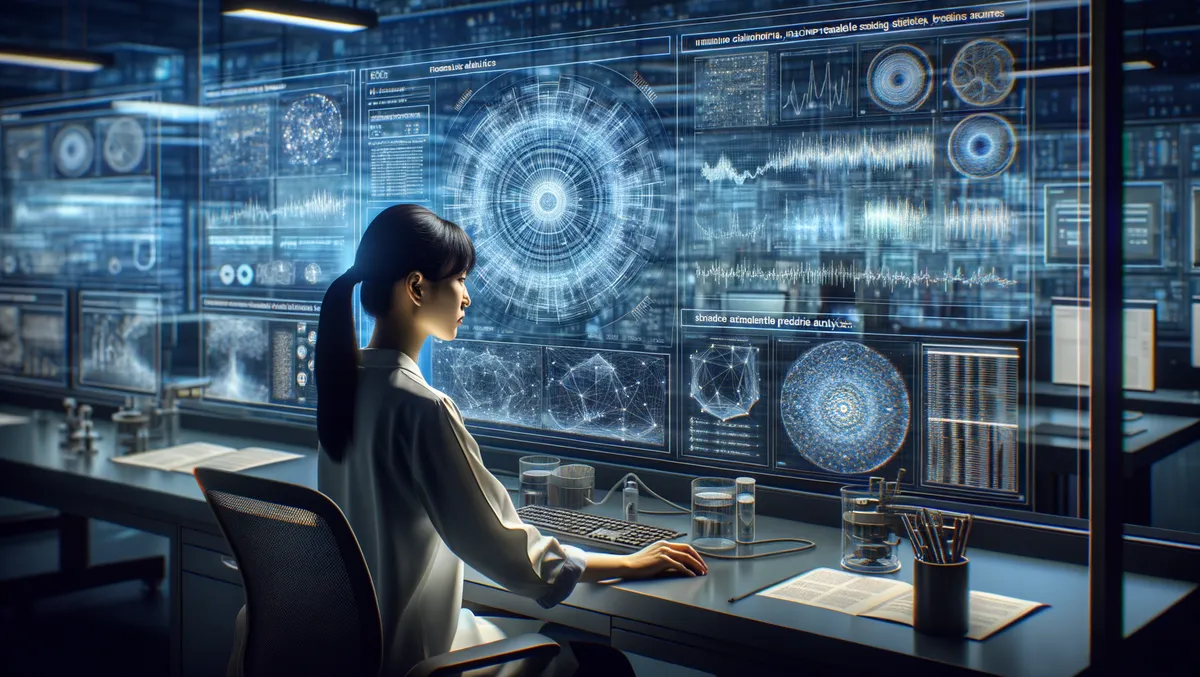
Elsevier, a global expert in scientific information and analytics, has launched Datasets, a new research product to assist a range of industries including life sciences, engineering, chemicals, and energy. The product utilises generative AI and predictive analytics technologies, addressing the frequent challenge of data scientists having to dedicate significant time to source quality data for well-trained AI models.
Datasets speeds up the digital transformation process by providing comprehensive, machine-readable data derived from trusted academic sources. With the ability to be fully integrated into private and secure computational ecosystems, its implementation helps safeguard intellectual property. The product aims to accelerate innovative thinking and business-critical decision-making processes in sectors heavy in research and development.
Elsevier’s Datasets have a range of potential applications. These vary from determining the appropriate material for the development of a product by accessing sources such as Elsevier's 271 million chemical substance records, to predicting drug efficacy and toxicity using advanced neural networks. Additionally, businesses can uncover company-wide expertise in specific disciplines through Elsevier's 1.8 billion cited references and 17 million author profiles.
"R&D-intensive businesses are excited by the possibilities of generative AI, predictive modelling and other areas at the vanguard of data science," commented Gino Ussi, President of Corporate Markets at Elsevier. "Nevertheless, to deliver high-quality analytics and well-trained AI models, data scientists must still devote much of their time to sourcing quality data. This process is time-consuming due to the sheer volume of research literature and carries risk if the data is not from a trusted and validated source."
The Datasets can be used to power a variety of applications in sectors including pharmaceuticals, chemicals, energy, materials, and technology, by integrating data from Elsevier into secure computational ecosystems.
This includes custom applications and third-party tools. For example, in drug discovery, Datasets can be deployed for selecting and confirming lead candidates and assisting with protein-ligand binding QSAR modeling. Moreover, pharmaceutical companies might find Datasets beneficial for pharmacovigilance, clinical trial design, and to inform market access strategies.
Materials science and informatics can benefit from Elsevier Datasets by selecting the appropriate material for a given application or product based on property prediction and relevant datasets analysis.
Across all disciplines, Datasets enables KOL identification and rising star selection, along with predictive modelling, knowledge graph creation, enterprise search, business intelligence dashboards, and algorithm and neural network training, the company states.
"Elsevier’s Datasets provide data from the world's largest source of scientific information to be embedded within R&D and business workflows. Research teams can also benefit from the expert support of our Professional Services team of data scientists, bioinformaticists, ontologists and domain specialists to help them scale and accelerate their data-led projects," added Mark Sheehan, Vice President of Data Science, Life Sciences at Elsevier.