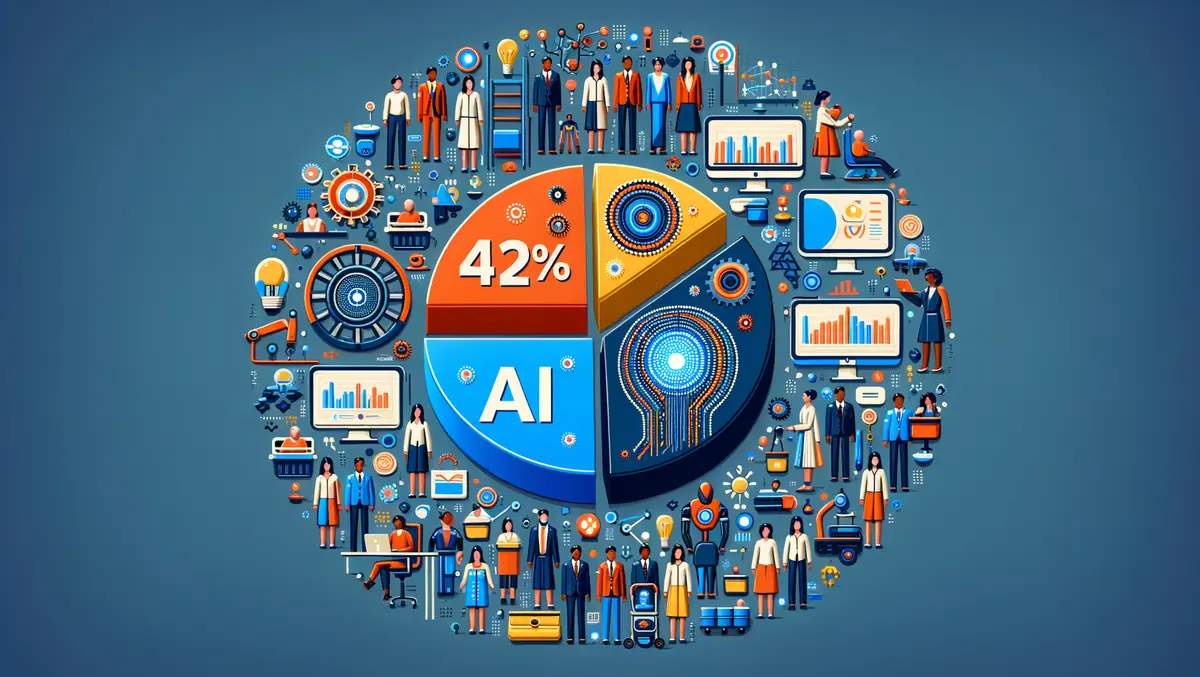
IBM research: 42% of companies adopt AI, success hinges on workforce trust
New research from IBM divulges that 42% of enterprises have released artificial intelligence (AI) deployments into their businesses, with a further 40% currently investigating its use. As this digital transition continues to gather pace, industry experts point out that the successful adoption of new machine learning (ML) and AI models hinges critically on workforce acceptance and trust.
Bartek Roszak, Head of AI at STX Next, a world expert in IT consulting, speaks to the issue. The technology innovation expert details his top five recommendations for deploying accessible and comprehensible machine learning models. His first tip emphasises the necessity of being forthright with all those inevitably influenced by the new technologies within a company's ecosystem.
"Ensure that you communicate implementation of any new technologies clearly to all those impacted well ahead of time," advises Roszak. "This means being upfront about how this will affect everyone's day-to-day and clear on what the intended impact of the models are."
Honing data quality and assessing the viability of a project via proof of concept is Roszak's second suggestion. "A model is only as good as the data it's trained on," states Roszak. "Ensure that it is fit for purpose, free of bias and be transparent so that end users are aware of what is being used to generate the necessary outputs."
Importantly, Roszak outlines that the ML process doesn't end with model deployment. On the contrary, it's just the beginning. Continuous adaptations and further implementation steps are necessary to fine-tune models and ensure their effective operation. Continuous monitoring, regular updates, and compliance with privacy regulations are fundamental facets of this process.
Roszak's fourth tip calls for education within the workforce. Employees should be made familiar with ML outputs and have a sound understanding of how to best leverage them. "Being able to understand a model's outputs and utilise them to their advantage is crucial to success," says Roszak, advocating for training sessions and encouraging queries and suggestions from employees.
Roszak's final suggestion asks companies to strike a balance in their approach to ML and AI technologies. Dispelling fears of job redundancies, Roszak asserts that many machine learning models have been developed to relieve humans from mundane tasks or to function in areas beyond the scope of human abilities rather than replace human roles. "Fear of job cuts is widespread across a number of industries at the moment, but ultimately, good models still need skilled people to interpret and understand outputs before putting them into practice," concludes Roszak.
Roszak's insights and recommendations illuminate the importance of honest communication, quality data, continuous shaping of models, employee education, and balance in the successful implementation of AI and ML models in businesses.