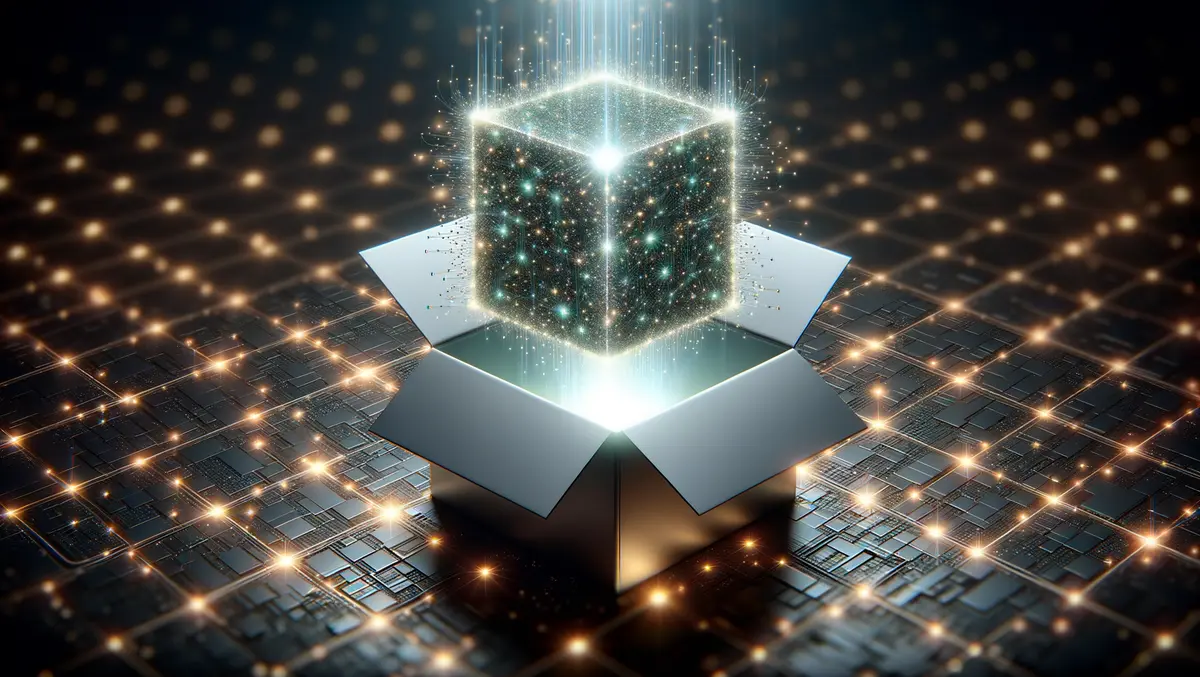
Siemens unveils Catapult AI NN to boost AI accelerator design
Siemens Digital Industries Software has introduced Catapult AI NN, a new software designed to streamline the development of AI accelerators for system-on-chip (SoC) designs. This solution enables software engineers to translate AI models created in Python into silicon-based implementations, facilitating faster and more power-efficient execution compared to traditional processors.
The Catapult AI NN software utilises hls4ml, an open-source package for machine learning hardware acceleration, in conjunction with Siemens' Catapult High-Level Synthesis (HLS) software. This unique combination addresses the specific requirements of machine learning accelerator design for power, performance, and area on custom silicon. The development of this robust framework was carried out in close collaboration with Fermilab, a U.S. Department of Energy Laboratory, alongside other leading contributors to hls4ml.
Mo Movahed, Vice President and General Manager for High-Level Design, Verification, and Power at Siemens Digital Industries Software, highlighted the inefficiencies associated with the manual process of converting neural network models into hardware implementations. He noted that this process is often time-consuming and prone to errors, especially when creating and verifying various hardware accelerator variants tailored to specific performance, power, and area needs. Catapult AI NN aims to mitigate these challenges by allowing scientists and AI experts to leverage industry-standard AI frameworks seamlessly and synthesise these models into hardware designs optimised for power, performance, and area (PPA).
As AI and machine learning tasks increasingly migrate from data centres to various consumer and medical devices, the demand for "right-sized" AI hardware is growing. This shift necessitates minimising power consumption and cost while maximising end-product differentiation. Traditionally, machine learning experts have been more adept at working with tools like TensorFlow, PyTorch, or Keras, rather than synthesizable C++, Verilog, or VHDL, thereby limiting their ability to accelerate machine learning applications in ASIC or SoC implementations.
The hls4ml initiative, intended to bridge this gap, generates C++ from neural networks described in popular AI frameworks such as TensorFlow, PyTorch, or Keras. This C++ code can then be deployed for FPGA, ASIC, or SoC implementation. Catapult AI NN further extends the capabilities of hls4ml to ASIC and SoC design, offering a dedicated library of specialised C++ machine learning functions tailored for ASIC design. These functions enable designers to optimise PPA by making latency and resource trade-offs across alternative implementations derived from the C++ code.
Panagiotis Spentzouris, Fermilab Associate Lab Director for Emerging Technologies, emphasised the stringent edge AI constraints in particle detector applications. He stated that the collaboration with Siemens allowed the development of Catapult AI NN, a synthesis framework capable of leveraging the expertise of scientists and AI experts without necessitating extensive ASIC design expertise.
Catapult AI NN offers the potential to revolutionise AI development, fostering new possibilities for AI and machine learning software engineers. By automating the implementation of neural network models for optimal PPA concurrently during the software development process, Siemens aims to usher in a new era of efficiency and innovation in AI development. The software is currently available for early adopters and will be more broadly accessible towards the end of 2024.