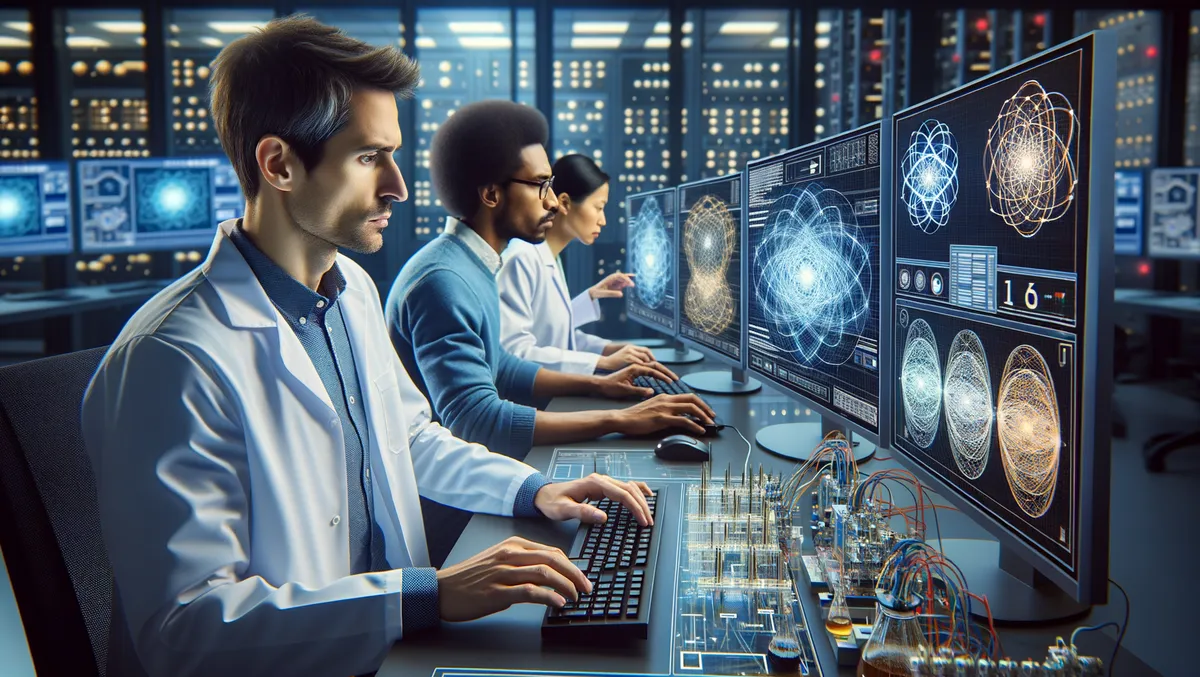
Zapata Computing, also known as Zapata AI, an industrial generative AI company, has announced their recent research in quantum-enhanced generative AI has been published in the respected journal Nature Communications.
This groundbreaking research, titled "Synergistic pretraining of parametrized quantum circuits via tensor networks", explores how quantum circuits could serve to enhance and expand the capacities of classical generative AI.
Christopher Savoie, the CEO and co-founder of Zapata AI, lauded the researchers involved, stating, "We are extremely proud of the talented researchers who contributed to this groundbreaking work."
He went on to elaborate on the potential advantages quantum techniques could bring to enterprise generative AI applications, explaining that, "Quantum techniques can bring tremendous advantages to enterprise generative AI applications, and this research shows how we can make the most of the resources we have today to realise those advantages."
Savoie further discussed the future prospects, challenging the conventional 'quantum versus classical' narrative by asserting, "It is no longer a question of quantum vs. classical, but rather how the two can be used synergistically together to get better results, faster." He concluded by expressing his eagerness to apply the newfound research to their collaborations with enterprise customers.
The work expands on Zapata AI's steadily proliferating portfolio of quantum techniques for generative AI. Quantum techniques can potentially provide significant advantages, notably in dealing with computations within enterprise applications.
These include compressing massive, computation-intense models, accelerating expensive and time-consuming calculations, and generating a more diverse spectrum of high-quality outputs for generative AI.
Jacob Miller, a Quantum Research Scientist at Zapata AI, highlighted the study's focus on combining the complementary qualities of quantum and classical computing to achieve superior results.
"Our work combines the complementary strengths of quantum and classical computers to reach better results than either type of hardware on its own," said Miller.
He clarified a common misconception, expressing, "People often think that quantum and classical technologies are in competition with each other, but we show that classical methods can actually help overcome a major limitation in the optimisation of quantum devices."
Another significant contributor, Jing Chen, a Senior Quantum Scientist at Zapata AI, explained the critical role played by tensor networks, traditionally used in classical algorithms, in forming a bridge to quantum algorithms.
Chen stated, "Our approach fosters collaboration, leveraging the strengths of classical and quantum methods to address complex problems more effectively." The research promises to enhance both fields and offers a solution to the frustrating challenges of barren plateaus in quantum computing.